“Using collaborative filtering, I noticed how my movie app started suggesting films I actually enjoyed, just by tracking what other users with similar tastes had watched. It felt like the app was learning my preferences without me having to manually rate every movie.”
“Collaborative Filtering Is A Classification Of Software That” Collaborative filtering is a method that recommends items based on patterns in user behavior and shared preferences. It helps suggest options by finding similarities between users with comparable interests.
Introduction To Collaborative Filtering Is A Classification Of Software That
Collaborative filtering is a classification of software that recommends items by examining user interactions and preferences. It analyzes patterns to identify similarities among users, making collaborative filtering a classification of software that enhances recommendation accuracy.
This method can suggest movies, products, or songs, demonstrating that collaborative filtering is a classification of software that adapts to diverse needs. By learning from user behavior, collaborative filtering is a classification of software that ensures personalized experiences for each individual.
What Is Collaborative Filtering Software?
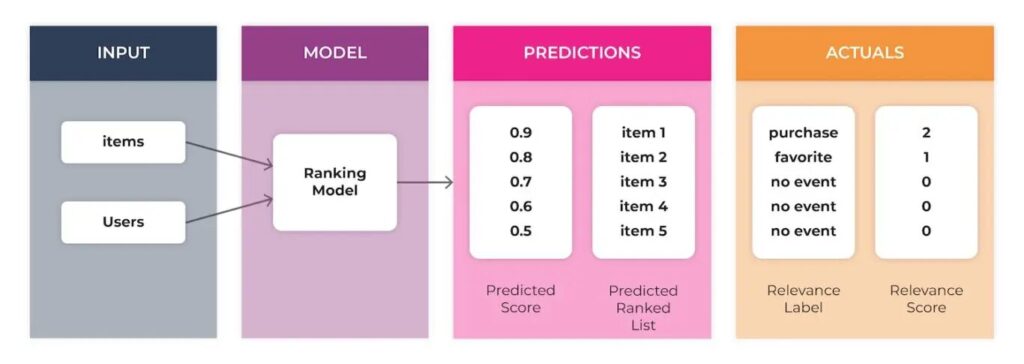
Collaborative filtering software provides personalized recommendations by examining user behavior and preferences. It identifies patterns and similarities among users with shared interests. This method is often utilized to suggest products, movies, or music tailored to individual tastes. Ultimately, it aims to enhance user experience through relevant and engaging suggestions.
How Collaborative Filtering Works?
1. User-Based Collaborative Filtering:
User-based collaborative filtering identifies individuals with similar interests to predict a user’s preferences. It suggests items that those like-minded users have engaged with or found enjoyable.
2. Item-based Collaborative Filtering:
Item-based collaborative filtering focuses on the relationships between items, recommending products based on their similarities rather than user preferences. This approach highlights items that are often liked or purchased together, enhancing the discovery process for users.
Types Of Collaborative Filtering
1. Memory-Based Collaborative Filtering:
Memory-based collaborative filtering generates recommendations by analyzing the complete dataset of user preferences. It identifies similarities between users or items based on previous interactions to predict what a user might enjoy.
2. Model-Based Collaborative Filtering:
Model-based collaborative filtering employs machine learning algorithms to forecast user preferences by analyzing their past behaviors. It creates a model from user data, allowing for efficient and tailored recommendations.
3. Hybrid Methods:
Hybrid methods integrate various recommendation techniques, like collaborative filtering and content-based filtering, to enhance accuracy. This combination results in more tailored and dependable suggestions for users.
Applications Of Collaborative Filtering
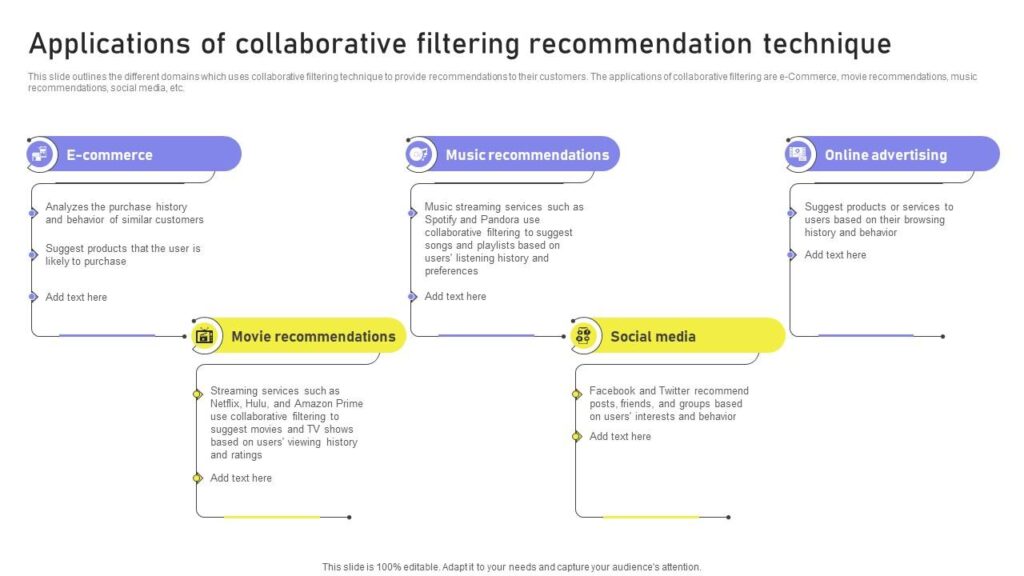
1. E-Commerce Recommendations:
- Personalized Shopping Experience: E-commerce platforms use algorithms to analyze user behavior and suggest products that match individual preferences, enhancing the shopping experience.
- Cross-Selling Opportunities: Recommendations often include items frequently bought together, encouraging customers to purchase additional products they may not have considered.
- Dynamic Updates: These systems continuously adapt based on user interactions, ensuring that the recommendations stay relevant as trends and preferences change.
- Increased Engagement: By providing tailored suggestions, e-commerce sites can boost user engagement and drive higher conversion rates, leading to increased sales.
2. Streaming Services:
- Wide Selection: Streaming services offer a vast library of movies, TV shows, and music, allowing users to access content on-demand.
- Personalized Recommendations: These platforms use algorithms to suggest content based on viewing history and user preferences, enhancing the user experience.
- Accessibility: Users can enjoy streaming services on various devices, including smartphones, tablets, smart TVs, and computers, making it convenient to watch anywhere.
- Original Content: Many streaming services produce exclusive shows and films, attracting subscribers with unique and engaging content that can’t be found elsewhere.
3. Social Media And Content Curation:
“Social media platforms leverage collaborative filtering to curate content, tailoring feeds based on user interactions and preferences. This personalized approach enhances user engagement by showcasing relevant posts, articles, and videos that align with individual interests.”
Advantages Of Collaborative Filtering
- Personalized Recommendations: Tailors suggestions based on individual user preferences, leading to a more engaging experience.
- Discoverability: Helps users find new items or content they might not have encountered otherwise, broadening their choices.
- Community Insights: Leverages the collective opinions and behaviors of a user base, providing a richer understanding of trends.
- Adaptability: Continuously improves recommendations as more user data is gathered, becoming more accurate over time.
- Simplicity: Offers a straightforward way to recommend items without needing detailed information about each user or item.
Challenges And Limitations
Collaborative filtering faces several challenges, such as the “cold start” problem, where new users or items lack enough data for accurate recommendations. It can also struggle with sparsity, as user interactions may not be sufficient to identify meaningful patterns.
Additionally, this method may inadvertently reinforce biases by recommending popular items, limiting exposure to less mainstream options. Finally, privacy concerns arise since user behavior data is often required, raising issues about data security and user consent.
Improving Collaborative Filtering Techniques
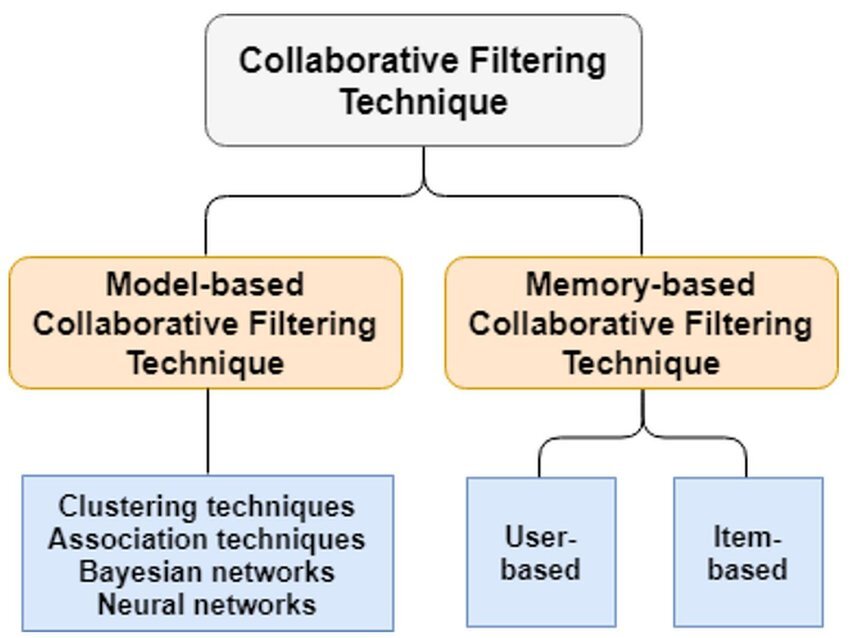
1. Data Preprocessing:
Data preprocessing involves cleaning and organizing raw data to ensure it is suitable for analysis. This step typically includes removing duplicates, handling missing values, and normalizing data formats, making it easier to extract valuable insights from the dataset.
2. Incorporating Contextual Information:
Incorporating contextual information into collaborative filtering enhances recommendations by considering factors like location, time, and user mood. This approach allows for more relevant suggestions, making the recommendations feel more tailored to the user’s current situation and needs.
3. Using Hybrid Models:
- Enhanced Accuracy: Hybrid models combine various recommendation techniques, such as collaborative and content-based filtering, to improve prediction accuracy and relevance for users.
- Broader Coverage: By integrating multiple data sources, these models can recommend items even when user data is limited, reducing issues like cold starts.
- Diverse Recommendations: Hybrid approaches provide a richer set of suggestions, helping users discover both popular items and niche options they may enjoy.
Real-World Examples Of Collaborative Filtering
1. Amazon’s Recommendation System:
Amazon’s recommendation system uses collaborative filtering and machine learning to suggest products based on users’ browsing and purchase histories. This approach personalizes the shopping experience, helping customers discover items that align with their preferences and interests.
2. Netflix’s Movie Recommendations:
Netflix employs collaborative filtering to evaluate viewer preferences, recommending movies that similar users have liked. This method allows users to uncover new films that align with their individual tastes, improving their overall viewing experience.
3. Spotify’s Music Suggestions:
Spotify uses collaborative filtering to analyze user listening habits and preferences, allowing it to recommend songs and artists tailored to individual tastes. This approach helps users discover new music that aligns with their interests, enhancing their overall listening experience.
Collaborative Filtering Is A Classification Of Software That Qui
Collaborative filtering is a software classification that focuses on recommending items based on user interactions and preferences. It analyzes patterns of behavior among users to identify similarities, which helps generate personalized suggestions.
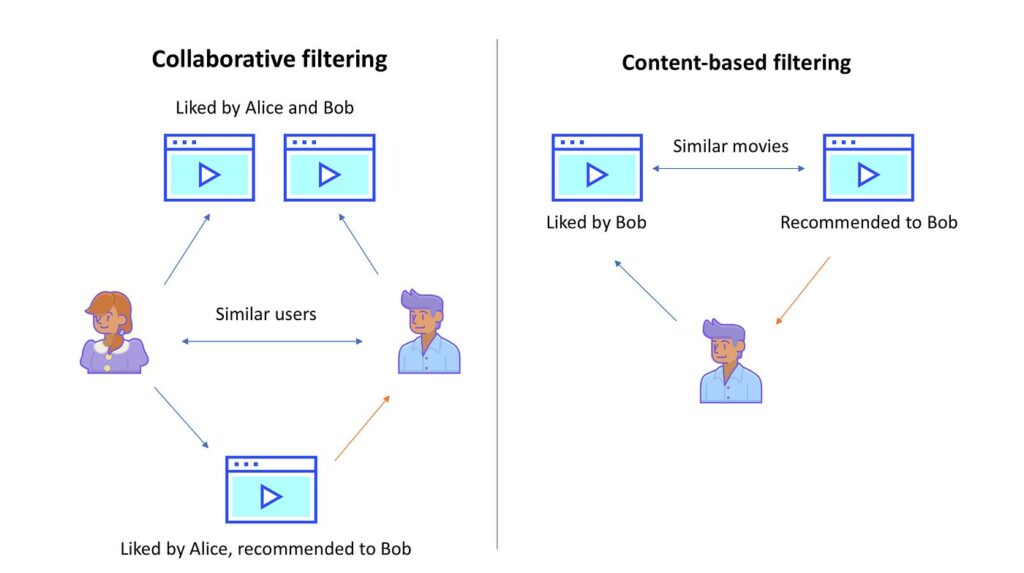
This technique is widely used in various applications, including streaming services, e-commerce, and social media platforms. By leveraging collective user data, collaborative filtering enhances the overall experience, making it easier for individuals to find content or products they will enjoy.
FAQ’s
1. Is Collaborative Filtering Classification Or Regression?
Collaborative filtering is primarily a classification method, as it categorizes items based on user preferences and behaviors to make recommendations. However, it can also involve regression techniques when predicting user ratings or preferences for specific items.
2. Which Activity Demonstrates The Use Of Collaborative Filtering?
A common activity that demonstrates collaborative filtering is when a streaming service recommends movies based on what similar users have watched and enjoyed. This approach helps users discover new films they might like by learning from the preferences of others with comparable tastes.
3. Can collaborative filtering work with small datasets?
Collaborative filtering struggles with small datasets because it relies on sufficient user interactions to make accurate recommendations.
4. What is the difference between user-based and item-based collaborative filtering?
User-based collaborative filtering focuses on finding similar users to recommend items, while item-based filtering recommends items based on similarities between the items themselves.
5. How does collaborative filtering handle privacy concerns?
Many platforms anonymize user data and implement strict data protection measures to address privacy concerns while using collaborative filtering.
Conclusion:
Collaborative filtering is a powerful tool that enhances user experiences by providing personalized recommendations based on collective preferences. By analyzing patterns in user behavior, it helps individuals discover items they might enjoy, whether in music, movies, or products.
Despite its challenges, such as data sparsity and cold starts, it remains widely used across various platforms. Ultimately, collaborative filtering enriches the way users interact with content, making their choices more relevant and enjoyable.